What is Sound Source Localization
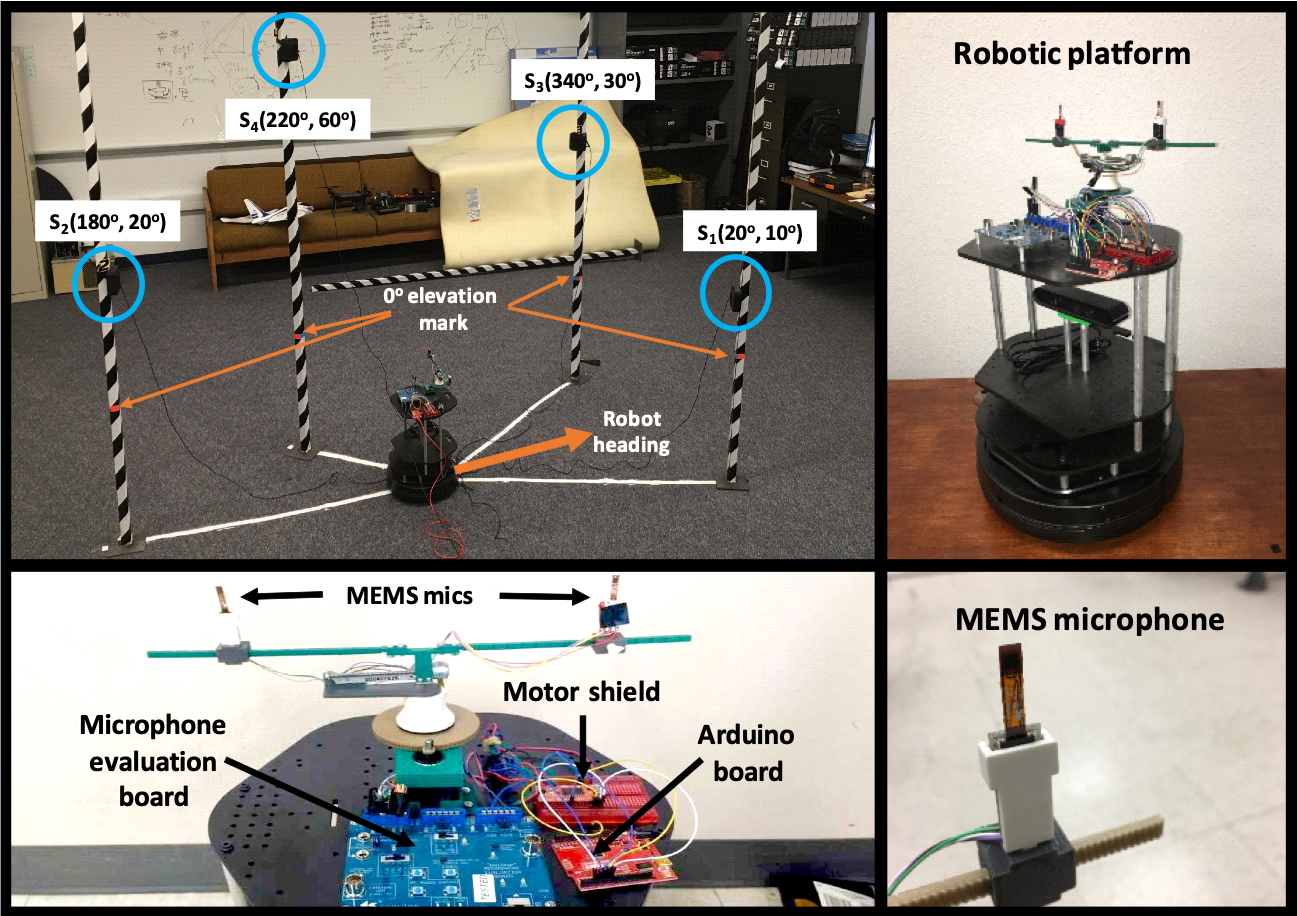
Sound Source Localization is the attempt to replicate humans' ability to determine what direction a sound is originating from and at what distance that sound is originating from. This research emulates human ears by using the delay between a sound wave hitting one ear and then the next ear to determine what direction the sound is coming from. This time delay is called the Interaural Time Difference.
Three-Dimensional Sound Source Localization for Unmanned Ground Vehicles with a Self-Rotational Two-Microphone Array
This paper presents a novel three-dimensional (3D) sound source localization (SSL) technique based on only Interaural Time Difference (ITD) signals, acquired by a self-rotational two-microphone array on an Unmanned Ground Vehicle. Both the azimuth and elevation angles of a stationary sound source are identified using the phase angle and amplitude of the acquired ITD signal. An SSL algorithm based on an extended Kalman filter (EKF) is developed. The observability analysis reveals the singularity of the state when the sound source is placed above the microphone array. A means of detecting this singularity is then proposed and incorporated into the proposed SSL algorithm. The proposed technique is tested in both a simulated environment and two hardware platforms, ie, a KEMAR dummy binaural head and a robotic platform. All results show the fast and accurate convergence of estimates.
Realtime Active Sound Source Localization for Unmanned Ground Robots Using a Self-Rotational Bi-Microphone Array
This work presents a novel technique that performs both orientation and distance localization of a sound source in a three-dimensional (3D) space using only the interaural time difference (ITD) cue, generated by a newly-developed self-rotational bi-microphone robotic platform. The system dynamics is established in the spherical coordinate frame using a state-space model. The observability analysis of the state-space model shows that the system is unobservable when the sound source is placed with elevation angles of 90 and 0 degree. The proposed method utilizes the difference between the azimuth estimates resulting from respectively the 3D and the two-dimensional models to check the zero-degree-elevation condition and further estimates the elevation angle using a polynomial curve fitting approach. Also, the proposed method is capable of detecting a 90-degree elevation by extracting the zero-ITD signal ’buried’ in noise. Additionally, a distance localization is performed by first rotating the microphone array to face toward the sound source and then shifting the microphone perpendicular to the source-robot vector by a predefined distance of a fixed number of steps. The integrated rotational and translational motions of the microphone array provide a complete orientation and distance localization using only the ITD cue. A novel robotic platform using a self-rotational bi-microphone array was also developed for unmanned ground robots performing sound source localization. The proposed technique was first tested in simulation and was then verified on the newly-developed robotic platform. Experimental data collected by the microphones installed on a KEMAR dummy head were also used to test the proposed technique. All results show the effectiveness of the proposed technique.
Multi-Sound-Source Localization Using Machine Learning for Small Autonomous Unmanned Vehicles with a Self-Rotating Bi-Microphone Array
While vision-based localization techniques have been widely studied for small autonomous unmanned vehicles (SAUVs), sound-source localization capabilities have not been fully enabled for SAUVs. This paper presents two novel approaches for SAUVs to perform three-dimensional (3D) multi-sound-sources localization (MSSL) using only the inter-channel time difference (ICTD) signal generated by a self-rotating bi-microphone array. The proposed two approaches are based on two machine learning techniques viz., Density-Based Spatial Clustering of Applications with Noise (DBSCAN) and Random Sample Consensus (RANSAC) algorithms, respectively, whose performances were tested and compared in both simulations and experiments. The results show that both approaches are capable of correctly identifying the number of sound sources along with their 3D orientations in a reverberant environment.